Accenture - AI & the Future of Corporate Services & Sustainability
Hello my wonderful new Accenture friends and partners!
Thank you so much for allowing me to share the time with you for our discussion on AI and the Future of Corporate Services! Now that the event is over, as promised, I have made this page with a few things which may be helpful.
– a downloadable PDF SUMMARY of the most important slides from my talk
– the AI NOTES from the talk from Otter.ai
– the QUESTIONS that we didn’t get to in our Q&A
If you want to here more from me in the future, you can subscribe here.
Dan Chuparkoff
A.I. & Innovation Keynote Speaker
CEO, Reinvention Labs
AI Educator & Innovation Expert from
Google, McKinsey, & Atlassian
dan@chuparkoff.com | 1.312.869.9777
PDF SUMMARY from Dan’s AI talk
Download the 3 page high resolution PDF here:
AI NOTES from Dan’s Keynote
*These notes were created automatically by Otter.ai. Transcription errors or mistakes have not been fixed
in order to demonstrate the current state of AI notes.
Summary
Dan Chuparkoff, a leading AI expert, discussed the transformative impact of AI on work, emphasizing its role as an assistant rather than a co-pilot. He highlighted the evolution of AI from basic text generation to complex tasks like image description and language translation. Chuparkoff explained AI's reliance on consensus and the importance of human oversight to mitigate bias. He noted AI's potential to enhance communication and collaboration globally, reducing language barriers. Despite AI's benefits, he stressed the need for humans to focus on problem-solving, discovery, and imagination, leveraging AI to handle routine tasks and improve efficiency.
Action Items
[ ] @Dan Chuparkoff - Dan to provide the AI-generated notes from the presentation.
[ ] Follow up on the questions about the sustainability and energy impact of AI.
Outline
Introduction and Meeting Kickoff
Speaker 3 introduces the AI session, mentioning the recording and the external speaker, Dan Chuparkoff.
Dan Chuparkoff thanks Lisette and mentions his background at Google and his current focus on AI.
Dan begins his presentation by discussing the impact of AI and comparing it to the discovery of fire.
Dan explains the importance of understanding AI and its role in transforming work.
Understanding AI and Its Applications
Dan uses a humorous analogy to explain AI's role in generating content and making predictions.
He describes the process of AI generating images and the importance of specificity in AI applications.
Dan shares his personal journey and how technology has transformed his life and career.
He outlines the major technological transformations over the past few decades and their impact on collaboration and information sharing.
AI as a Prediction Machine
Dan explains the concept of generative pre-trained transformers (GPT) and their role in AI.
He conducts a live demonstration to illustrate how AI predicts the next word in a sentence.
Dan emphasizes the importance of understanding AI's confidence levels and the limitations of AI-generated answers.
He discusses the role of AI in various applications, such as email drafting and content generation.
The Evolution of AI Models
Dan provides a historical overview of AI models, starting with Google's first transformer model in 2017.
He explains the evolution of AI models, including Google BERT and OpenAI's GPT series.
Dan highlights the significant advancements in AI, particularly with the release of ChatGPT and its rapid adoption.
He discusses the impact of increased training data on AI's accuracy and capabilities.
AI Tools and Their Applications
Dan introduces various AI tools, including ChatGPT, Copilot, Claude, Gemini, and Llama.
He explains the unique features and applications of each tool, such as data privacy and integration with enterprise software.
Dan emphasizes the importance of choosing the right AI tool for specific needs and applications.
He discusses the broader landscape of AI tools and their role in transforming software development and collaboration.
AI in the Workplace
Dan explains how AI can be used to enhance workplace efficiency and collaboration.
He discusses the role of AI in generating better emails, drafting strategy documents, and improving communication.
Dan emphasizes the importance of using AI as an assistant rather than an automator.
He provides examples of how AI can be integrated into various industries and roles to improve productivity and decision-making.
The Role of AI in Problem Solving
Dan explains the hierarchy of human expertise and the role of AI in different types of work.
He discusses the importance of problem-solving, discovery, and imagination in human expertise.
Dan emphasizes that AI is best suited for predictive and repetitive tasks, while humans excel in creative and complex problem-solving.
He highlights the potential of AI to free up human time for more strategic and innovative work.
AI and Global Collaboration
Dan discusses the potential of AI to enable global collaboration by breaking language barriers.
He provides a live demonstration of AI-generated translations in multiple languages.
Dan emphasizes the importance of language accessibility and how AI can make the world a smaller place.
He discusses the broader implications of AI for global collaboration and the creation of a more equitable world.
Addressing AI Bias and Ethical Considerations
Dan addresses concerns about AI bias and the importance of human oversight in AI decision-making.
He emphasizes the need for regular reviews and approval layers to ensure AI outputs align with human values and priorities.
Dan discusses the role of feedback in improving AI models and reducing bias.
He highlights the importance of creating a world that values diversity and equity to produce less biased AI.
Closing Remarks and Q&A
Dan concludes his presentation and opens the floor for questions.
Speaker 3 moderates the Q&A session, addressing questions about AI's impact on education, bias, and the future of AI.
Dan provides insights and answers questions, emphasizing the importance of human oversight and the evolving role of AI in the workplace.
The session ends with expressions of gratitude and appreciation for Dan's presentation and insights.
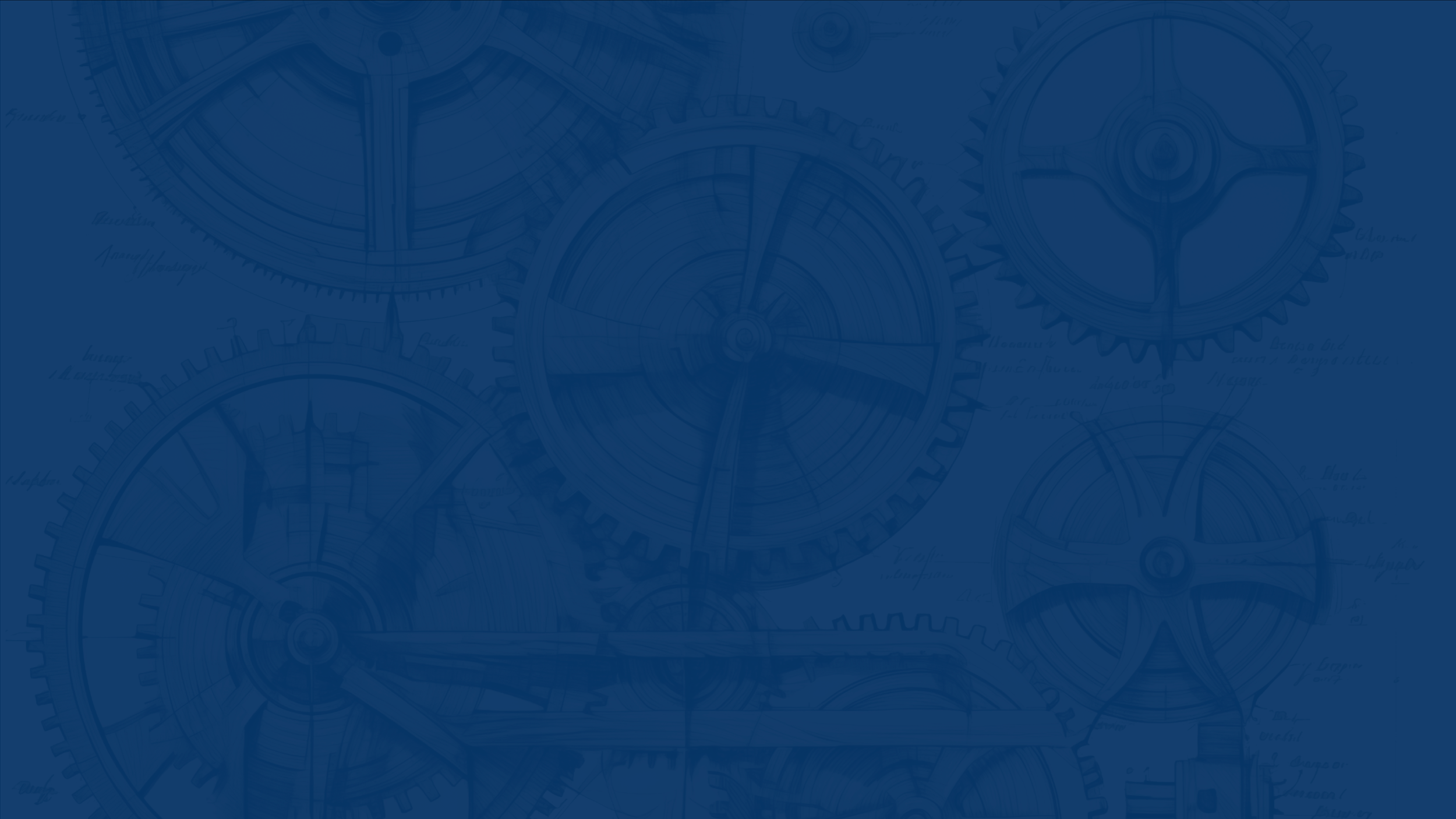
These are the QUESTIONS
that we didn’t have time for
Humble opinions on some of those outstanding questions. If you want to discuss more, reach out anytime. You can find me on LinkedIn here: https://www.linkedin.com/in/chuparkoff
What advancements are coming that reduce the computing power and energy required to make the AI function (thinking sustainability)
Energy usage of AI training and the invoking of AI is certainly an issue that needs to be mitigated. But I think there are two things that will mute this problem significantly over time.
The first is that some amount of the training of AI right now is working to understand foundational things such as the rules of grammar and logic, translations between English and Spanish, and more. Some of these things don’t need to be part of future training runs. This should reduce the time and energy it takes to train new versions of the algorithm.
The second is that most users are using AI for free – or for very small subscription fees ($9/mo). Because using AI is virtually free right now, people are using it without any concern for cost. At some point though, the cost of training and running the algorithms will get passed on to the consumer. In that future world, people will likely constrain their use of AI in relation to the cost. And this will certainly curb use of the models significantly. At that moment it will also likely drive the AI training organizations to make sure that they are constraining use of energy.
In a world where we are plenty of fake news and wrong information, how can we ensure AI systems are trained with good quality data and not with wrong/interested data provided by people? Are data reviewers working on that? How can we ensure these reviews are not compromised with their believes or biases?
This is pretty important: We can’t! It will always be your responsibility as an AI user to investigate the recommendations that AI gives you and decide if those answers come from legitimate sources and that those answers align with your experience, morals, and ethics. It’s critically important to understand here, that there are not really data-reviewers as you describe in your question. GPT 4o was trained with 13 trillion bits of data. It would take a reasonably-sized team 126,000 years, to review 13 trillion pieces of data for accuracy and bias. The answers that come from AI just come from the Internet. And the Internet is filled with an equal amount of Experts, Fools, and Fiction Authors. Please don’t ever put AI on Autopilot. AI needs your review, every single time.
Are there any standard safeguards to ensure AI systems are not only effective and efficient but also fair, transparent, and accountable, especially in the context of preventing bias, ensuring data privacy, and addressing the risk of algorithmic manipulation?
The most important safeguard is to ensure that AI isn’t making decisions for you, you are. AI is an information aggregator. It’s been trained off of data that comes from wildly, conflicting opinions and that will always be true. This is similar to the previous question. AI’s job is to pull the information that exists in the world together and to show it to you so that you can figure out how to make the right decision for your team and so that you can figure out the courses of action that align with your objectives, beliefs and morals. The information that exists in the training data is filled with bias and unfairness. And it always will be. So it’s important to look at the information that comes from it, and apply a healthy skepticism upon it before you move forward on any course of action.
Can AI conduct data analytics, providing insights, trends and predictions based on past data
It depends on what you mean when you say, “AI” here. Data Scientists, Analysts, and Developers have been using Machine Learning and Predictive Analytics to look for trends in data, find patterns, and draw conclusions for a long time now (maybe 25 years). If you have a data science team, or some team of analysts and developers, then they might be doing this already. But if when you say “AI”, if you mean “ChatGPT”, “Claude”, or “Gemini”, then you should proceed cautiously. Generative AI, like the AI we see in ChatGPT is built upon a language model (not a mathematical one). So uploading a spreadsheet into ChatGPT and asking it hard questions is likely going to fail a lot of the time. Current Language Model AI benchmarks show reliable performance on elementary math only. More complex mathematical operations are only handled correctly about 65% of the time or less. AI will describe what it sees, and it excels at language, but it’s still not really that great for now at math. Also be intimately familiar with the privacy policy of the AI tools you’re using when you start feeding it your data. Different AI tools have different policies on what happens to data that has been ingested. If you’re interested in exploring Mathematical AI a little further, make sure to check out Wolfram Alpha.
Just some of my thoughts! If you want to discuss more, reach out anytime. You can find me on LinkedIn here: https://www.linkedin.com/in/chuparkoff